Understanding Bounding Boxes in Data Annotation: A Comprehensive Guide
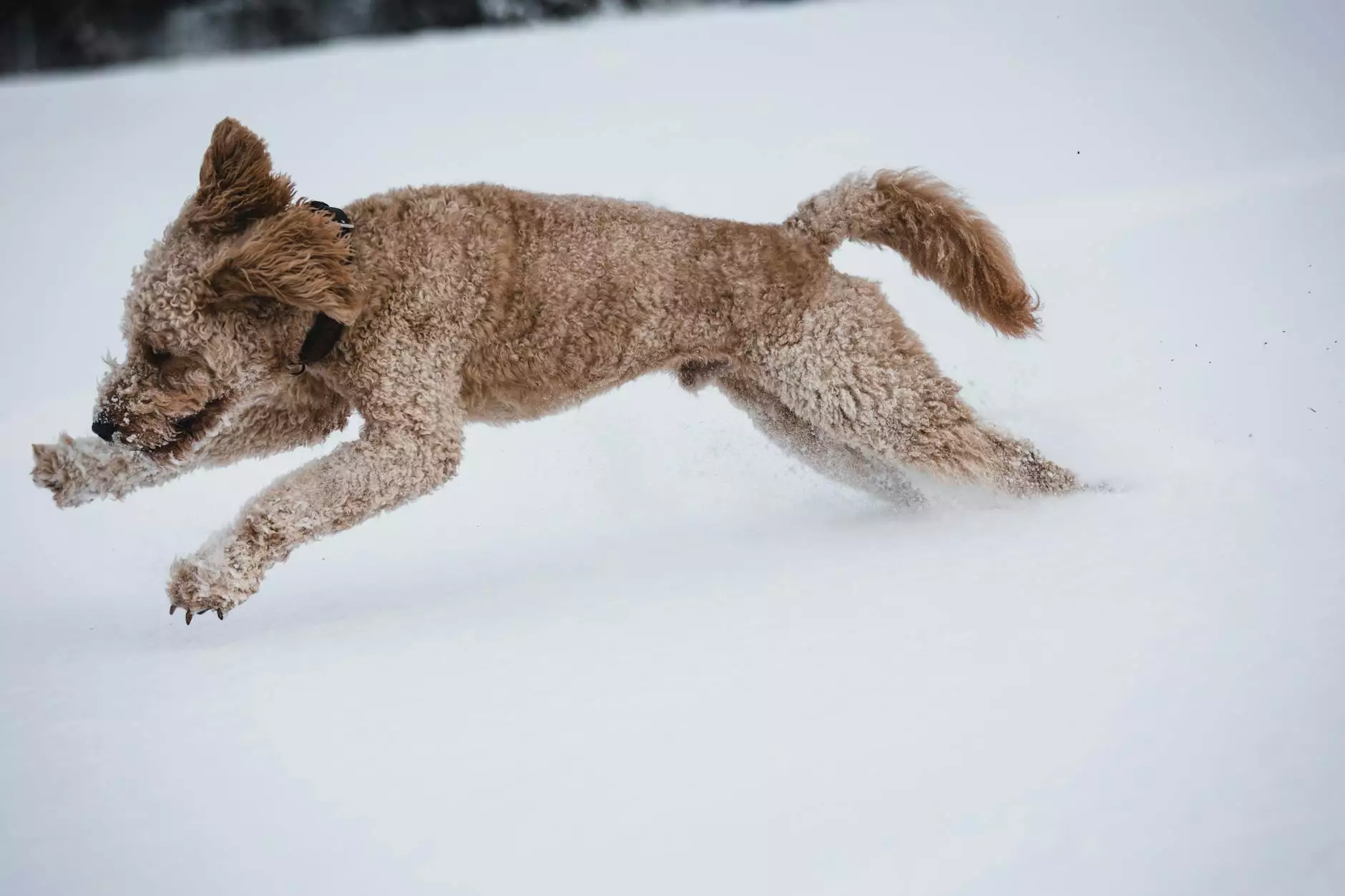
Bounding boxes play a crucial role in the realm of data annotation, particularly in the fields of computer vision and graphic design. As a visual representation of objects within an image, bounding boxes serve as a vital framework for understanding and training artificial intelligence (AI) systems. In this article, we will explore the significance of bounding boxes, their applications, and how businesses can leverage them effectively.
What Are Bounding Boxes?
Bounding boxes are rectangular boxes that frame an object of interest within an image or video. Used extensively in computer vision, they help isolate specific items, enabling algorithms to detect and learn from them. Each bounding box is defined by its top-left and bottom-right coordinates, creating a clear demarcation of the area where the object resides.
Technical Definition
From a technical standpoint, a bounding box is represented by four coordinates: (x_min, y_min, x_max, y_max). These coordinates correspond to the top-left and bottom-right corners of the rectangle that encapsulates the object. This simplicity allows for easy calculations and processing, making bounding boxes a favored choice in various AI applications.
The Importance of Bounding Boxes in Data Annotation
Bounding boxes are essential in data annotation for several reasons:
- Object Recognition: They help algorithms identify and locate objects within a larger image. For instance, in autonomous vehicles, bounding boxes allow the system to identify pedestrians, vehicles, and road signs.
- Training AI Models: Accurately annotated data is crucial for training machine learning models. Bounding boxes provide a clear dataset for the model to learn from, ultimately improving its predictive capabilities.
- Efficiency and Scalability: Automated tools can generate bounding boxes quickly, facilitating the scaling of data annotation projects. This is particularly useful in industries like e-commerce, where vast numbers of images require annotation.
Applications of Bounding Boxes
Bounding boxes are utilized across various sectors:
1. Computer Vision
In computer vision, bounding boxes are paramount for tasks such as:
- Object Detection: Algorithms are trained to detect objects in real-time, which is vital in applications like security surveillance and robotics.
- Image Segmentation: While pixel-wise segmentation provides even finer detail, bounding boxes still serve as a preliminary approach in categorizing visuals.
- Facial Recognition: Systems can use bounding boxes to isolate faces in an image for further analysis or recognition.
2. Graphic Design
In graphic design, bounding boxes aid in:
- Layout Design: Designers can use bounding boxes to create structured layouts, ensuring that elements are evenly aligned and proportionately placed.
- Responsive Design: Bounding boxes help in designing applications that adapt to various screen sizes, ensuring that images scale correctly without losing quality.
3. Medical Imaging
In the medical field, bounding boxes facilitate:
- Image Analysis: Bounding boxes help radiologists and AI models identify specific areas that may require closer examination, such as tumors in scans.
- Automated Diagnosis: AI systems, trained with bounding box annotations, can assist in diagnosing diseases by accurately locating anomalies in medical images.
The Process of Creating Bounding Boxes
Creating precise bounding boxes involves a systematic approach:
1. Image Selection
First, select the images or video frames that need annotation. The quality of these images directly impacts the effectiveness of the bounding box annotations.
2. Defining the Boundaries
Carefully use annotation tools to draw boxes around the objects of interest. It’s crucial to align the edges of the box closely to the contours of the object to avoid misleading AI interpretations.
3. Quality Assurance
Conduct thorough quality assurance checks to ensure that all bounding boxes are accurately annotated. This may involve cross-referencing with others or utilizing automated validation tools.
4. Scaling the Process
Once a productive workflow is established, scaling the annotation process becomes feasible. Utilizing technology and collaborative platforms can significantly enhance efficiency.
Why Choose KeyLabs.ai for Data Annotation?
At KeyLabs.ai, we understand the critical role that accurate bounding boxes play in successful data annotation. Here’s why we are the preferred choice for businesses:
1. Expertise in Data Annotation
Our team comprises experts in the fields of computer vision and machine learning, ensuring that your bounding boxes are created with precision and attention to detail.
2. Scalable Solutions
We offer scalable data annotation services that can grow with your project’s needs, allowing you to manage large datasets efficiently without compromising on quality.
3. Advanced Tools and Technology
Utilizing the latest technology, including AI-driven annotation tools, we can streamline the data annotation process, reducing time and cost while improving accuracy.
4. Customized Annotation Services
We understand that each project is unique. Our team collaborates with clients to develop tailored annotation strategies, including the specific use of bounding boxes, to meet their precise requirements.
Conclusion
In conclusion, bounding boxes are an indispensable component of data annotation that serve multiple industries, enhancing the capabilities of AI systems in object detection, image analysis, and design. As technology continues to advance, the significance of high-quality bounding boxes will only grow, emphasizing the need for efficient and accurate data annotation services.
At KeyLabs.ai, we are committed to delivering top-notch data annotation solutions that empower businesses to achieve their objectives while harnessing the power of accurate and reliable data.